Table Of Content
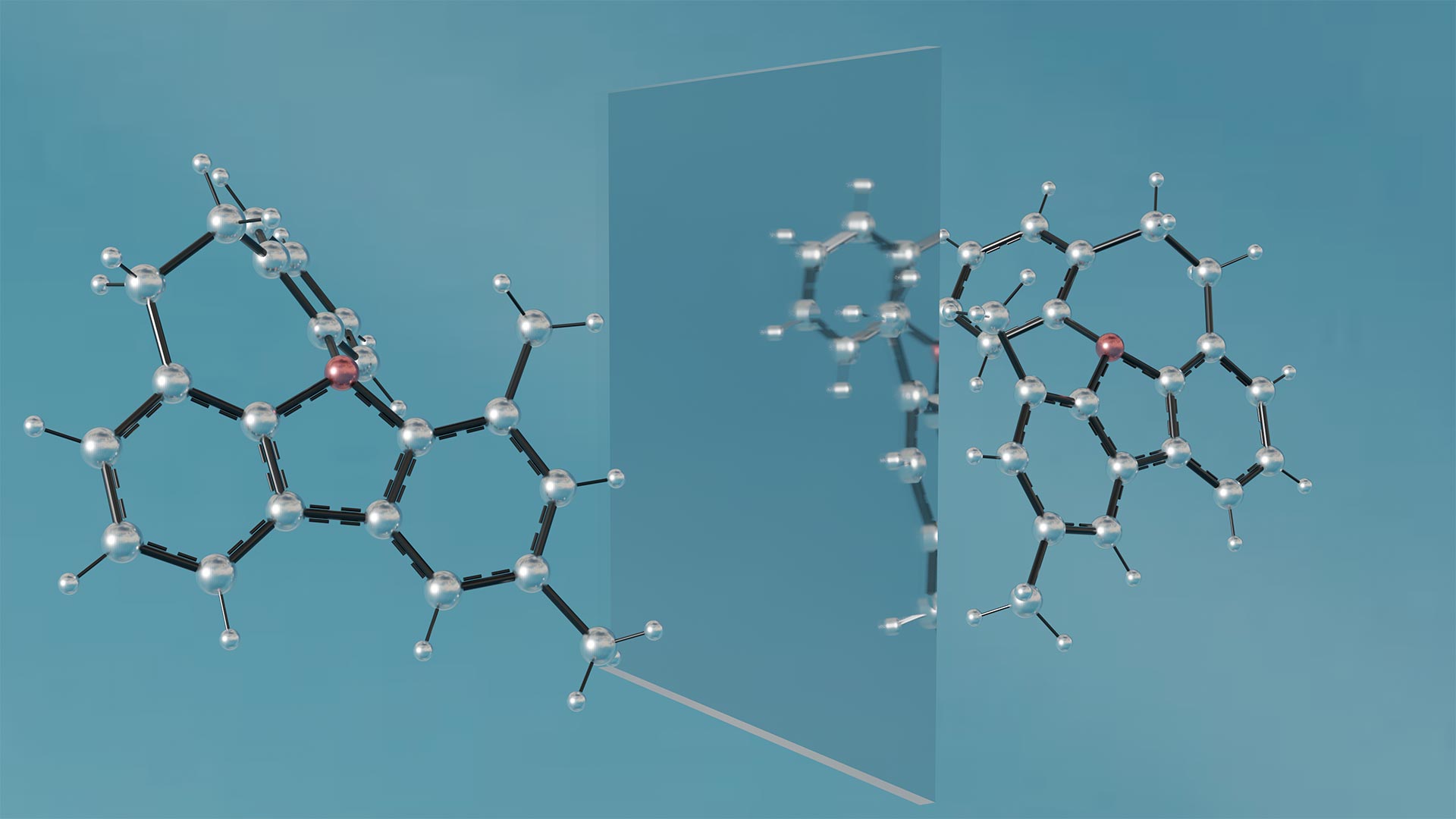
In the first test, the researchers’ model generated 100 percent chemically valid molecules from a sample distribution, compared to SMILES models that generated 43 percent valid molecules from the same distribution. Given a desired property, the model optimizes a lead molecule by using the prediction algorithm to modify its vector — and, therefore, structure — by editing the molecule’s functional groups to achieve a higher potency score. It repeats this step for multiple iterations, until it finds the highest predicted potency score. Then, the model finally decodes a new molecule from the updated vector, with modified structure, by compiling all the corresponding clusters.
Reconstruction of lossless molecular representations from fingerprints
This information is used by a readout phase to generate the feature vector for the molecule, which is then used for the property prediction. Closed-loop workflow for computational autonomous molecular design (CAMD) for medical therapeutics. It consists of data generation, feature extraction, predictive machine learning and an inverse molecular design engine.
Efficient enumeration-selection computational strategy for adaptive chemistry
Target labels for each molecule and its corresponding property are also generated by discretizing the target space into several bins. Correlations between edges often are decomposed into discrete sequenced representations during the generation trace. CGVAE [75] was incorporated two types of correlations including some known rules like valency rules as hard one and ring strain (adverse to cycles) as soft one. CGVAE was kept correlative to convert into SMILES with validity semantically. Notably, molecules with similar properties practically differed in the size of nodes and edges while generative models for molecular graphs like GraphVAE [70] did not take this into account. Samanta et al. [76] have proposed NEVAE aggregated features by distinct hops number.
Corwin Hansch dies at 92; scientist whose advances led to new drugs and chemicals
We study a broad array of problems, including chromosome dynamics, genome architecture, aging, neuroscience, developmental and evolutionary biology, microbial science, RNA science, and how genotype translates to phenotype. To tackle these problems we employ a diverse set of approaches, including biochemistry, genetics, genomics, computation, single molecule biophysics, cutting edge microscopy, and x-ray crystallography. Our experimental systems are equally varied, ranging from bacteria and yeast, to Drosophila, C. Elegans, zebrafish, Xenopus, mouse, human cell culture systems, and synthetic systems.
BIOVIA Discovery Studio - Dassault Systèmes
BIOVIA Discovery Studio.
Posted: Thu, 05 Oct 2023 21:39:19 GMT [source]
This process makes it possible to secure a group of potential new materials with properties that are not in the training data without significant changes in the shape of the molecular structure (Fig. 6). The predicted accuracy is low because of the relatively small number of molecules with very low S1 values in the overall data distribution. However, as the process continues to accumulate sufficient data, the predicted accuracy is likely to increase, and this would enable candidate materials with new values to be obtained. ECFP is a circular topological fingerprint that has been successfully applied to represent organic molecules in vector forms. The encoding function e(∙) uses a hash function to map the structural features of a molecule into a fixed-length ECFP vector representation. In this study, we employ an ECFP with a neighborhood size of 6 and a length of 5000.
The Milos collection is the fruit of Vondom's first collaboration with French designer Jean-Marie Massaud. An example of how simplicity and functionality can be combined to create an elegant and comfortable outdoor furniture collection. Y.K., S.K., and Y.S.C. designed the study, implemented the methodology, and wrote the manuscript.
Macrocyclization of linear molecules by deep learning to facilitate macrocyclic drug candidates discovery
Deep neural networks are divided into discriminative models and generative models. The discriminative models which reflect the difference between heterogeneous data are to find the optimal classification [7]. The generative models, modeling the prior probability, represent the similarity of congener data.
Masking in sequence-based models is introduced for maintaining local structural and functional properties like NEVAE, CGVAE. Graph-based generative models now play a dominant role in the molecular design due to the advantages of graphs and the development of graph neural networks, however, some challenges still remain. To our best knowledge, with the increases of nodes size, the total calculation will grow up the square of nodes number at least, which is difficult to acquire the precise likelihood.
Optimization strategy for molecular design
However, with coordinates, the representation of molecules is non-invariant, non-invertible, and non-unique in nature [35] and hence not commonly used in conventional machine learning. In addition, the coordinates by itself do not carry information about the key attribute of molecules, such as bond types, symmetry, spin states, charge, etc., in a molecule. Lately, various computer-aided techniques for designing materials, such as inverse designs, exhaustive enumerations, and molecular structure optimization models, have been proposed.
De Cao et al. [78] proposed GANs-based graph generative models, called MolGAN, utilized the likelihood free method and avoided the expensive graph matching procedure. Similar to ORGAN, MolGAN applied the policy gradient reward and WGAN with penalty gradient for small molecular graphs. Molecules are 3D multiconformational entities, and hence, it is natural to assume that they can be well represented by the nuclear coordinates as is the case of physics-based molecular simulations [62].
VAE-based models aim at maximizing the evidence lower bound (ELBO) of the likelihood with Kullback-Leibler divergence. Notably, the latent space of VAE for molecular generation is potentially operated such as controlling the specific properties and the training process is stable. However, reconstructing the training sets limits the ability of exploring in unknown chemical space. The past few decades have observed the arising of keen interest in computational methods especially with the emergence of deep learning.
In the increasing direction, the average S1 increases by approximately 60% for 50,000 training data; however, it increases by merely 45% for 10,000 training data. This seems to suggest that these results reflect the difference in the performance of the RNN models. The RNN model trained with a larger dataset learns more decoding rules and would decode fingerprints into more diverse molecular structures to meet the goals.
In this toolbar you can select from a number of elements, you can also pick an element from the periodic table using the last button.
No comments:
Post a Comment